Backtesting is a crucial aspect of algorithmic trading and involves simulating trading strategies using historical data. It enables traders to evaluate the efficacy of Forex trading strategies, identify profitable approaches, and refine future trades.
Backtesting involves using quantitative analysis, and market analysis tools to perform statistical analysis on trading simulations. It helps traders mitigate risks and identify trends that inform trade entry and exit strategies.
Successful trading requires discipline, risk management, and trade execution skills, which can all be optimized using backtesting. Trading software and automation platforms make backtesting more accessible and efficient.
It’s essential to remember that historical data analysis is not a guarantee for future performance. Hence, traders must backtest their trading strategies periodically, track performance metrics, and adjust their approaches accordingly. Using trading journals and reports to track trade history and scaling trades based on analysis and historical results can also enhance trading performance.
Soft 4 Fx
Soft 4 Fx is the most well known manual backtesting software in the forex industry. The lightweight tool bolts on to MetaTrader and allows you to use historical data to actually enter trades in the market and track your statistics.
- Cheap
- Quick To Setup
- Backtest Years Of Data In Minutes
- Easy To Use
A pro tip is to incorporate trading psychology principles, such as mindfulness, to optimize trading performance.
Number of Trades
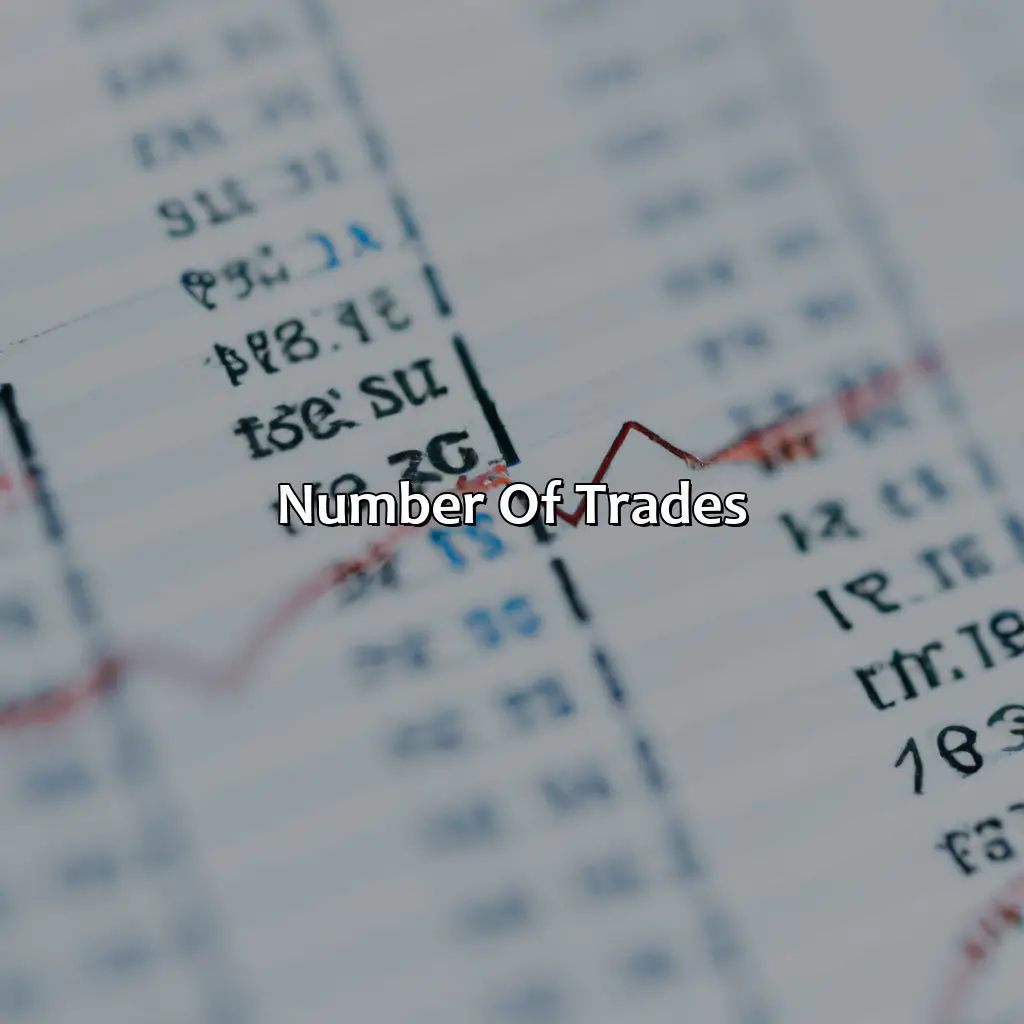
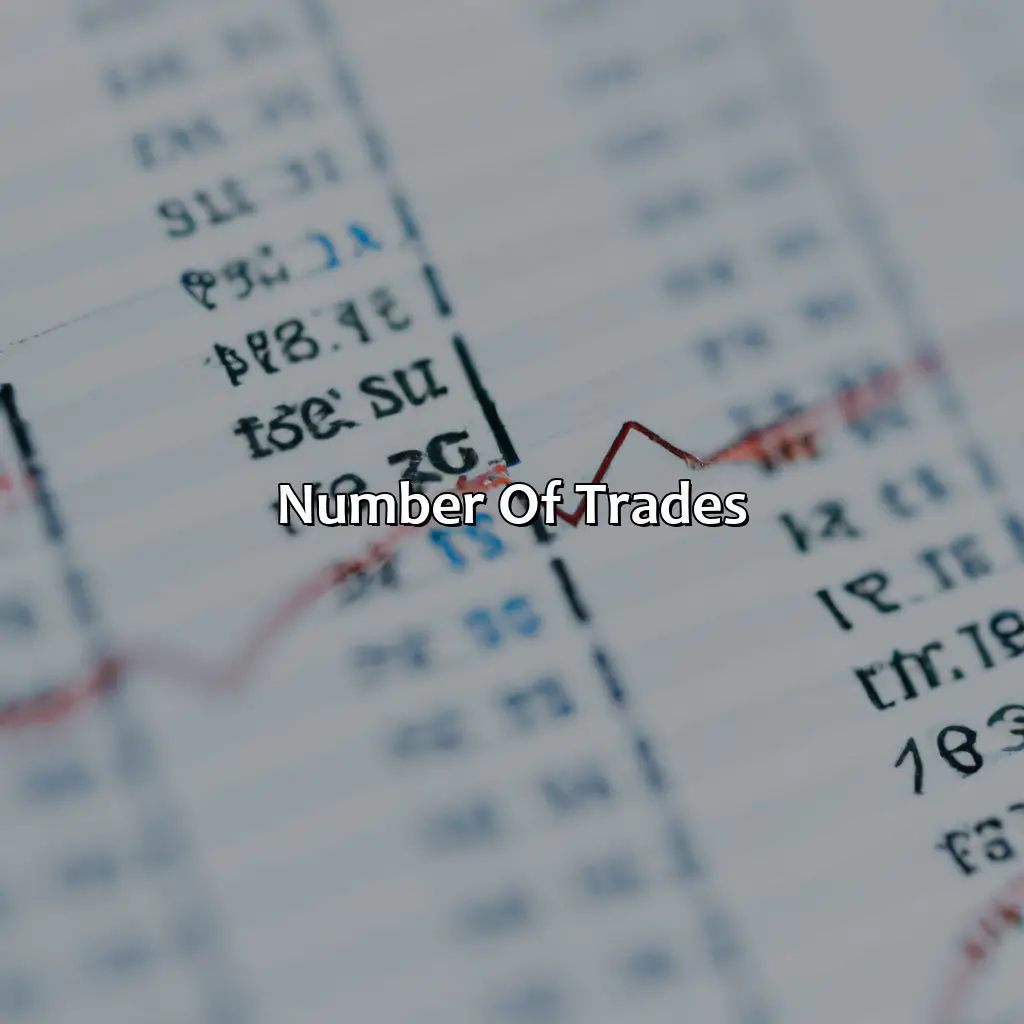
Photo Credits: forexbrokerreport.com by Zachary Lee
Analyzing optimal trade numbers for backtesting requires you to consider trade volume.
This section focuses on the importance of trade volume and factors that influence optimal trade numbers. Statistical and data analysis must be done too.
Significance of trade volume
The trade volume in backtesting plays an important role in indicating the overall significance of results. The number of trades executed is directly proportional to the potential accuracy of the strategy being tested. This metric influences the statistical significance of any analysis and thus, determines if it helps to discern patterns or simply occurs due to chance.
The significance of trade volume is paramount when it comes to determining if a strategy is effective or not. If there are too few trades incorporated in backtesting, even any random results may appear significant, leading to unreliable conclusions. Conversely, excessive trades may lead to overfitting and skewing results towards specific market conditions, reducing predicted performance during testing on out-of-sample data.
Understanding how much data will provide enough samples for testing a strategy is crucial when it comes to determining optimal trade sample sizes. Best practices encourage having at least 30 validated data points for better statistical power and stable findings concerning all types of trading strategies.
It’s essential to note that traders may continue adding more trades until meeting expectations but must keep in mind the law of diminishing returns. While additional trades reinforce reliability and serve as further validation points for a strategy’s efficiency, eventually, each additional unit fails to bring value proportionally.
Finally, traders need always to remain wary of overfitting their strategies within backtesting as this could lead them down paths that work solely with constrained markets or flukes instead of actual trends. By carefully selecting methods that do not fit noise levels and random events and then testing with a variety of instruments or timeframes can help avoid this problem while increasing accuracy overall.
Missing out on the benefits of appropriate trade volumes can be costly for traders who seek reliable strategies used for predicting future outcomes accurately. Therefore, we recommend implementing successful approaches based on adequate training data volumes rather than arbitrary criteria like minimum numbers required by some software systems most effectively nowadays.
Avoid trading like a cowboy, and consider factors like market volatility and risk management when deciding on the optimal number of trades.
Factors affecting optimal trade numbers
The number of trades executed can affect the accuracy and reliability of backtesting results. Selecting an optimal trade volume requires consideration of various factors. Key factors affecting optimal trade numbers include:
- trading frequency,
- market volatility,
- trading strategy complexity, and
- trading style.
These factors can influence the number of trades required to obtain statistically significant results and identify potential flaws in a trading system.
In addition to the aforementioned factors, it is essential to determine the adequate trade sample size. Statistical significance depends on the sample size and defines how confident we are that the result will be reproducible for future trades. Best practices suggest testing different sample sizes until achieving the smallest possible sample size without sacrificing accuracy and reliability.
In contrast, additional trades improve the validity of backtesting results by reducing variance errors, increasing the confidence level percentage, and providing more realistic backtested data. However, there is a point at which further trade additions offer no additional benefit as per the Law of Diminishing Returns.
Overfitting refers to excessively refining backtest parameters until they produce desired outcomes instead of accurately predicting future market movements; hence it must be avoided. Rigorously testing any optimization parameter before applying it allows traders to reach decisions that offer maximum generalization on all market conditions.
For example, silver traders adjusted their position sizing methods after finding out that too many open positions caused them to incur losses due to erratic price fluctuations. Factors such as this one involve choosing optimal trade volume for each strategy in every distinct market scenario while avoiding overfitting.
Choosing the right sample size in backtesting is like finding a needle in a haystack, but with statistics.
Determining the Adequate Trade sample size
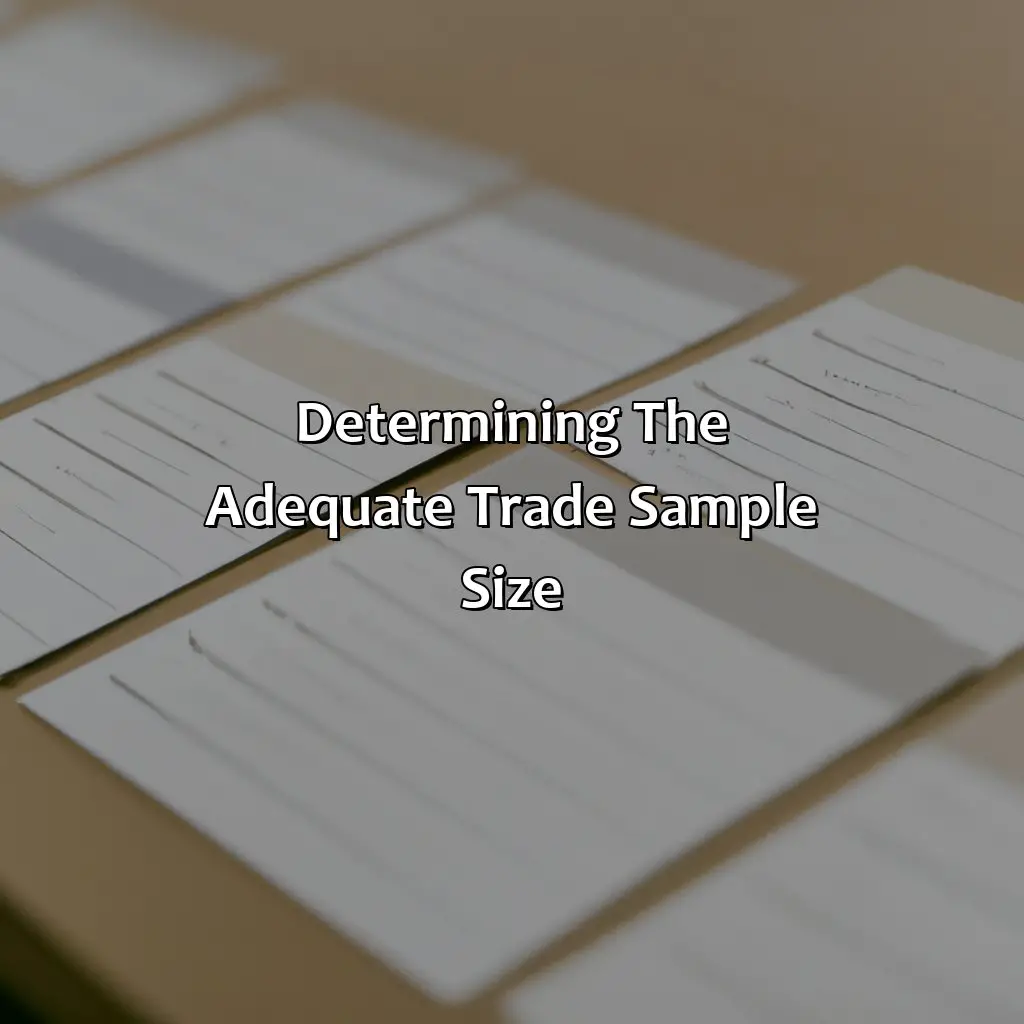
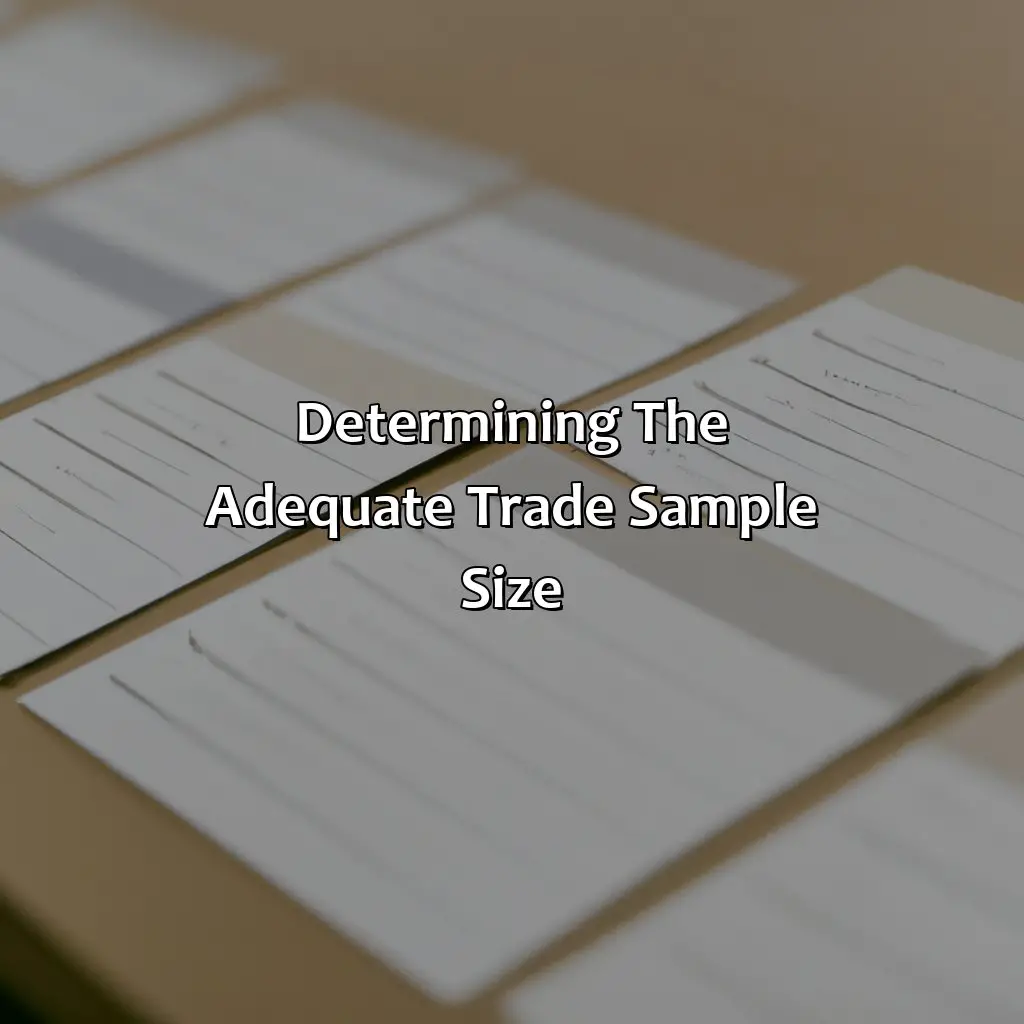
Photo Credits: forexbrokerreport.com by Michael Martinez
To pick the right trade sample size for backtesting with statistical importance, utilize the appropriate method. In this part on ‘Finding the Adequate Trade sample size’, we’ll investigate the importance of sample size in statistical analysis. Plus, you’ll uncover some of the top techniques for selecting a trade sample size that produces dependable results.
Statistical significance of sample size
For any backtesting analysis, determining an adequate sample size is crucial to ensure statistical significance. When it comes to the statistical significance of sample size, there are a few things to keep in mind.
- The larger the sample size, the more statistically significant the results. Consequently, if your sample size is too small, your results may not be reliable enough to make informed decisions.
Below is a table that demonstrates how different samples sizes can impact the reliability of results with varying levels of confidence intervals:
Sample Size | Confidence Interval |
---|---|
30 | +/- 10% |
50 | +/- 7% |
100 | +/- 5% |
200 | +/-2.5% |
As you can see from this table, as the sample size increases, there is a decrease in the margin of error with increasing levels of confidence intervals (assuming that other factors remain constant).
Another vital aspect when considering statistical significance is choosing an appropriate hypothesis test that accounts for both type I and type II errors. With trade samples of variable sizes comes a tradeoff between exploiting a higher resolution of data or reducing noise to mitigate overfitting concerns.
Interestingly, it’s worth noting that statistical significance doesn’t always equate practical significance. Although there may be significant differences between two groups concerning some outcome through backtesting calculations, whether or not these differences hold an operational difference is still open-ended without real-world experiences.
According to Investopedia [source], statisticians often say that conclusions are practically significant only if they have an effect on practical outcomes in addition to being statistically significant.
Finding the right trade sample size is like Goldilocks searching for the perfect bowl of porridge – not too big, not too small, just right.
Best practices for choosing trade sample size
When it comes to backtesting, determining the proper trade sample size is crucial. It ensures statistical significance and reliability of results. Here is a 3-step guide outlining the best practices for choosing trade sample size:
- Consider the Trading Strategy: Based on your trading strategy complexity, determine how many trades you need to have enough data points. For example, a simple trend-following strategy may require as few as 20 trades, whereas a more sophisticated strategy with several rules and filters may require at least 100 trades.
- Look at Historical Data Availability: Determine how much historical data you can obtain and use it to calculate the maximum number of trades possible during that period. This step will allow you to define what you can realistically expect from your backtesting efforts.
- Evaluate Available Computing Power: Depending on the computation needs of your strategies, evaluate the computational requirements and time needed for backtesting each trade in real-time, then determine if your computer resources are powerful enough.
It’s worth noting that market conditions are continuously changing; thus, using an adequate sample size does not guarantee future performance accuracy. Additional precautionary measures can also minimize risks and improve results. Choosing an optimal trade sample size is critical while backtesting to ensure adequate levels of statistical analysis; however, one should avoid overfitting their strategy by adjusting multiple variables often leading to inaccurate test results or outcomes that cannot be repeated or followed in real-life applications.
Don’t risk missing out on essential insights into your trading strategies – follow these best practices for choosing trade sample size! More trades can increase accuracy and reliability, but be careful not to fall victim to the law of diminishing returns.
The Impact of Additional Trades
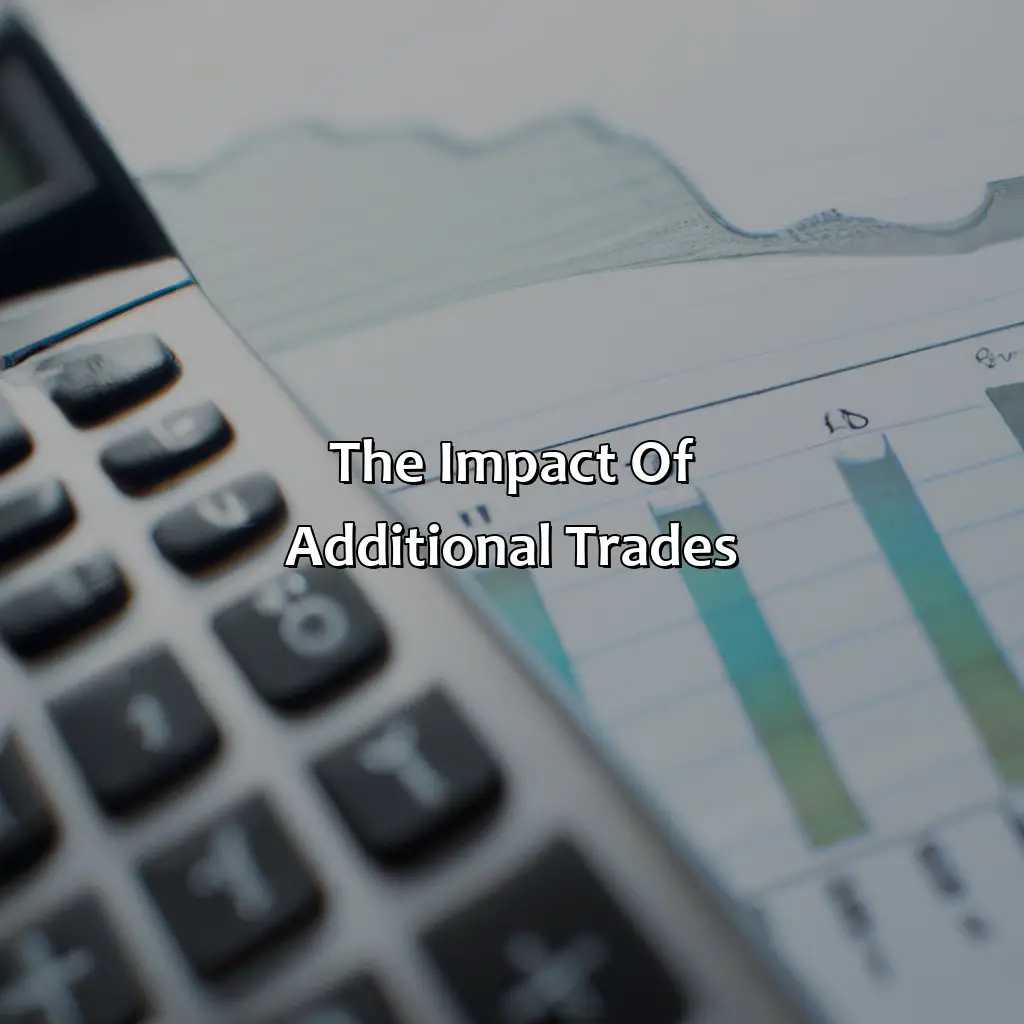
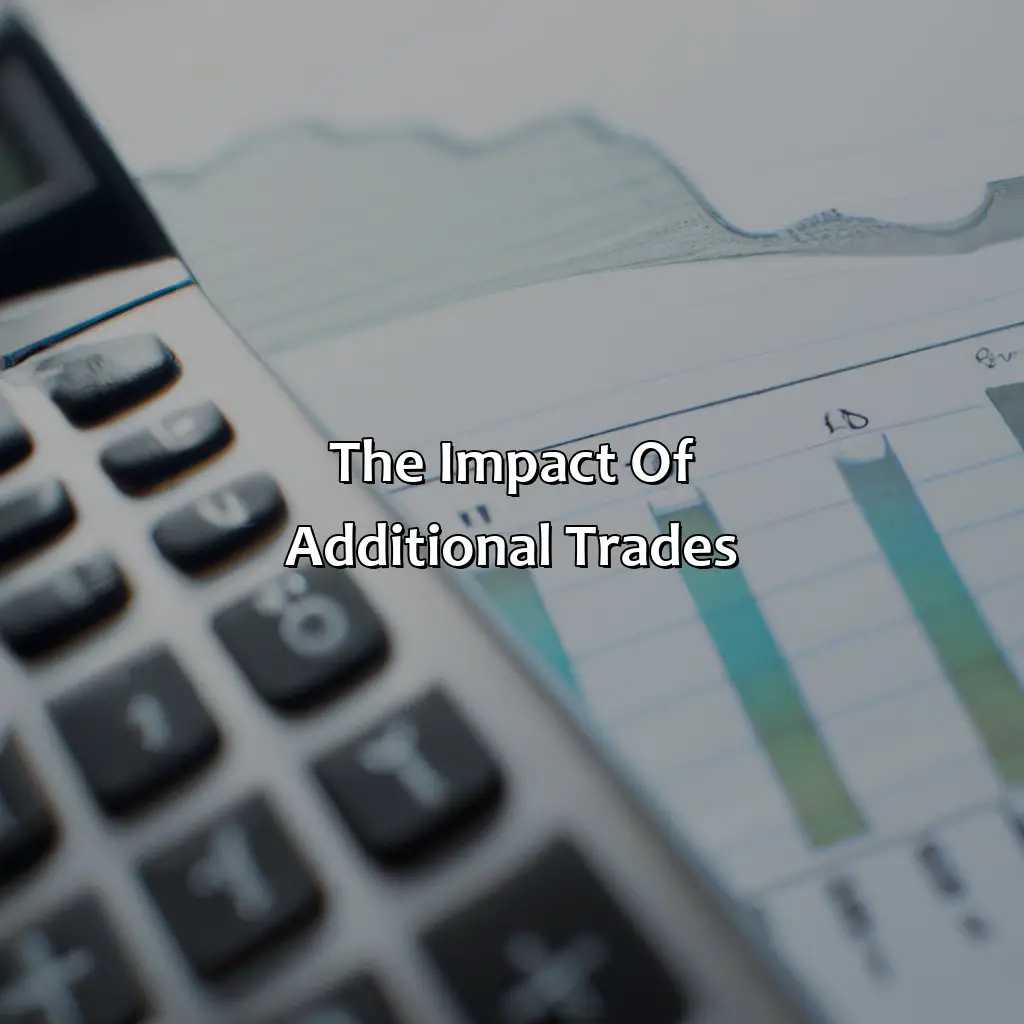
Photo Credits: forexbrokerreport.com by Wayne Lee
Achieving accurate and reliable backtesting results with more certainty? To analyze the impact of extra trades, Increase in Accuracy and Reliability and The Law of Diminishing Returns are here to help! These sub-sections offer the solutions.
Increase in Accuracy and Reliability
With more trades, the backtesting process experiences an increase in accuracy and reliability. The trade sample size influences how accurate the results are, impacting the dependability of the backtesting process.
Statistically significant sample sizes are crucial for market analysis, and increasing trade volume helps achieve that requirement. As a result, larger samples of trades provide greater reliability by increasing the number of data points used to determine results.
It’s essential to note that increasing trade volume does not always guarantee higher accuracy levels. In some cases, additional trades might not add any value since it could lead to diminishing returns.
To improve accuracy and reliability without adding unnecessary trades, traders can use machine learning models like Decision Trees or Random Forests that apply specific rules to avoid overfitting and prevent bias.
Overall, it is critical to maintain a balance between obtaining higher accuracy levels and avoiding overfitting while determining an adequate number of trades for backtesting purposes.
You can’t keep adding trades and expect better results, just like you can’t keep adding toppings to your pizza and expect it to taste better.
The Law of Diminishing Returns
As more trades are executed during the backtesting process, the law of diminishing returns becomes increasingly relevant. This principle states that while increasing inputs may initially increase output, there comes a point when additional inputs yield diminishing marginal returns. In other words, as more trades are added to the backtest, the benefits gained will eventually decrease at a certain point.
This law is crucial in determining the optimal number of trades for backtesting and ensuring that overfitting is avoided. As noted earlier, an inadequate sample size may lead to faulty conclusions that may not apply in real-world scenarios. However, adding an excessive number of trades can also lead to unnecessary complications and invalidate results.
To strike this balance, it is important to consider factors such as trade volume and statistical significance when deciding on the adequate amount of data needed for backtesting. An appropriate sample size should effectively account for randomness while still capturing relevant market trends statistically.
In light of this consideration, one suggestion would be to employ a staged approach where testing is gradually increased until achieving statistical significance but without reaching a point where results become convoluted or irrelevant. Another recommendation would be to use Monte Carlo simulations before drawing final conclusions from quantitative analysis provided by larger data sets.
Overall, it’s vital to keep in mind that adding too many trades can result in diminishing marginal returns. Therefore, traders should strike the optimal balance between increasing trade volume and retaining statistical reliability before arriving at any conclusion regarding trading strategies and systems.
Overfitting in backtesting is like trying on a shirt that’s too small and pretending it fits perfectly – it may look good now, but it’s bound to rip at the seams later.
Risks of Overfitting
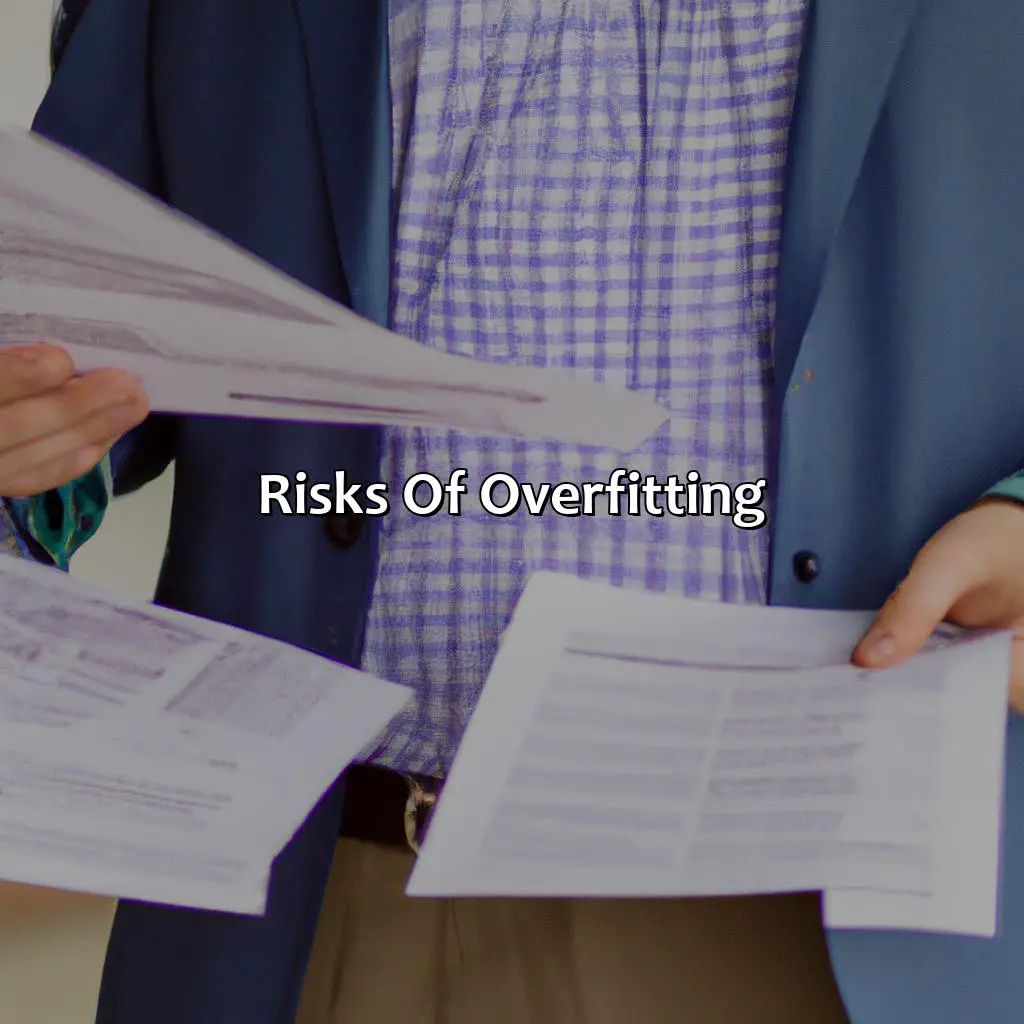
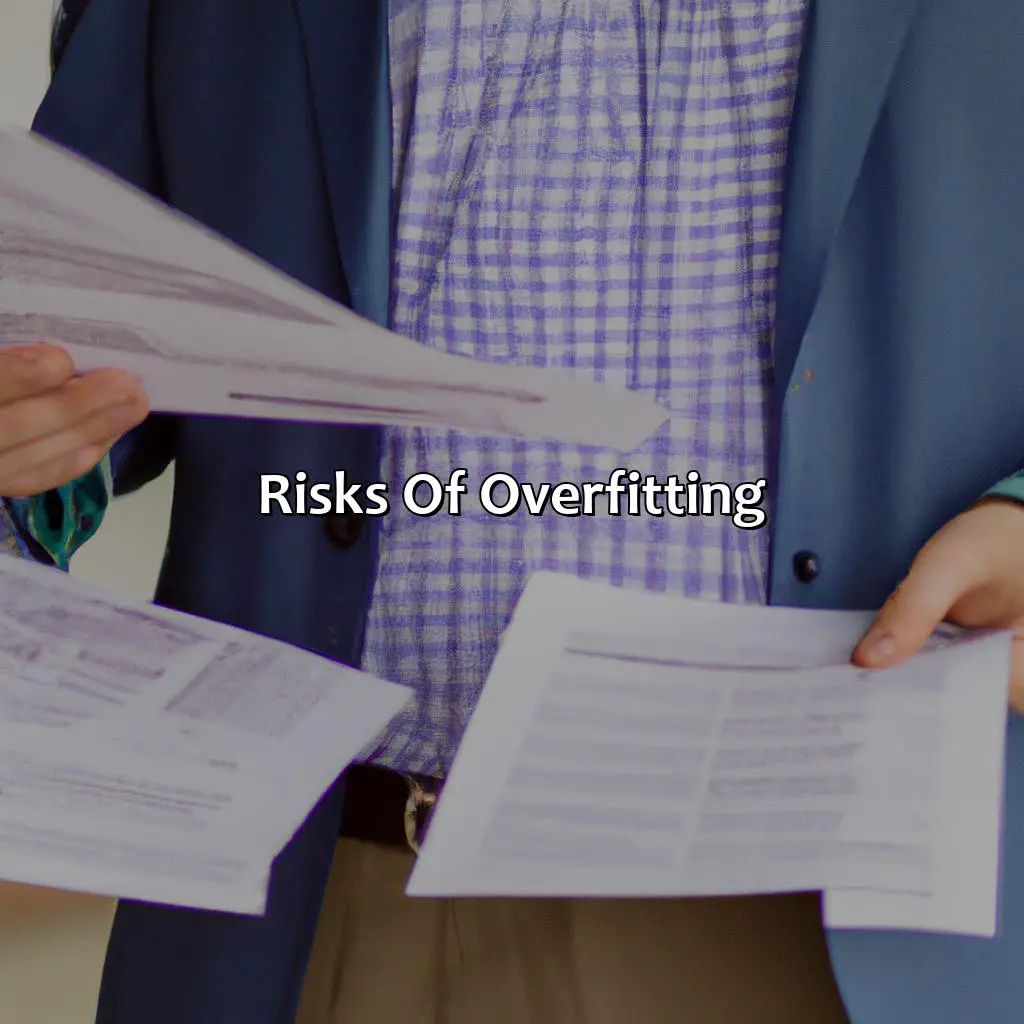
Photo Credits: forexbrokerreport.com by Bobby Carter
Stop overfitting when backtesting with small data sets by first understanding what overfitting is. To know the consequences and how it can hurt your results, check out ‘Consequences of Overfitting.’ Lastly, ‘How to Avoid Overfitting in Backtesting’ provides tips and tricks to avoid it.
Definition of Overfitting
Overfitting, in the context of backtesting, occurs when a trading strategy is tailored too closely to past market data. This results in excellent performance on historical data but poor performance in live trading. Overfitting arises from using too many parameters, curve fitting or choosing intervals arbitrarily to create ideal results on prior data. The most common reason that overfitting occurs is when traders try to fit complex models into small amounts of historical data. It’s important to note that over-optimization and overfitting can coexist.
A significant drawback of overfitted strategies is their inability to reproduce favorable results in live trading applications while maintaining stability and profitability under real-life conditions. Systems designed with too much emphasis solely on fitting historical figures often generate inaccurate signals lacking real-world insight and practical implementation.
Studies indicate that excessive trade numbers can serve as a red flag for overfitting and generate losses for an investment portfolio. As such, great care should be taken when choosing the best sample size during backtesting.
It must be noted that adequate sampling size varies depending on various factors including the degree of variance present in the dataset, level of confidence desired, and standard error margin. Choosing a sample size smaller than required could lead to inaccurate results while setting it larger than needed may prove time-consuming and ultimately wasteful.
One thing traders must also realize is that more trades don’t always translate into better systems. While additional trades offer promise for increasing efficiency, analysts need to consider the law of diminishing returns as well as associated risks before deciding on optimal quantities.
Overfitting affects accuracy substantially because traders run the risk of producing systems that suit historic events but are not efficient in theory or practice hence leading to suboptimal performances under real world circumstances.
According to Investopedia, “backtesting strategies will not always provide a guarantee for success since markets frequently transform through time.”
Overfitting in backtesting is like wearing rose-tinted glasses – it may look good, but it’s not a realistic view of the market.
Consequences of Overfitting
Overfitting can have dire consequences, leading to ill-informed decisions and erroneous investment strategies. The practice involves tweaking the parameters of a system to fit all available data, potentially leading to a model that performs poorly in the future. This can occur due to coincidental correlations, resulting in inaccurate predictions and loss of profits.
In such cases, overfitted models tend to generate seemingly favorable backtesting results but perform poorly in live trading scenarios. Such errors can be attributed to using small or inappropriate sample sizes during backtesting or relying on non-representative training data that are not reflective of real-world scenarios.
In addition, overfitting may cause excess trade volume, where an algorithm generates more trades than necessary due to its inability to generalize beyond training data. This increases transaction costs and may ultimately lead to unacceptable risk levels being taken when actual trading occurs.
Given these consequences, it is imperative that traders take adequate measures when developing investment models. They should avoid using insufficient data size or arbitrary modifications to parameters during backtesting workflows and instead rely on vigorous testing procedures that include out-of-sample validations where possible.
Backtesting without avoiding overfitting is like cooking with expired ingredients – it might seem fine, but the end result could be disastrous.
How to Avoid Overfitting in Backtesting
To prevent overfitting in backtesting, it is essential to avoid optimizing the trading model based on past data. Use out-of-sample testing and ensure that the model works well with data not used for tuning parameters. It’s crucial to maintain a healthy balance between minimizing bias and preventing overfitting by avoiding curve fitting and selecting robust models. Hyperparameter tuning should be achieved through cross-validation or other appropriate strategies to avoid over-optimizing the trading models.
It is also best to restrict the number of variables used in a model, reducing variables will reduce complexity and make it easier to identify predictors of the asset’s price movement that genuinely determines them. Moreover, it can lead to less overfitting since too many variables would probably lead to a more intricate algorithm producing complicated patterns from noise rather than directly actionable information.
A possible approach to limit overfitting is using regularization techniques like Ridge and Lasso regression. Regularization reduces the error by applying a penalty term proportional to magnitude effectively penalizing unnecessarily sophisticated models while prioritizing sensible one.
Pro Tip: One should keep checking their results against real market data regularly and adjust accordingly. Avoid over-optimization of models by continuously validating them against fresh market conditions or tweaking it periodically with new information instead of basing decisions solely on back-tested results.
Five Facts About Backtesting with 100 Trades:
- ✅ Backtesting with 100 trades is not enough to assess the effectiveness of a trading strategy. (Source: Investopedia)
- ✅ Extending the length of backtesting to 1000 trades or more can provide more meaningful results. (Source: Better System Trader)
- ✅ Backtesting should take into account market conditions and economic events during the testing period. (Source: TradingView)
- ✅ The quality of historical data used for backtesting can also affect the accuracy of results. (Source: EODHistoricalData)
- ✅ Backtesting should be combined with forward testing and paper trading to ensure the reliability of a trading strategy. (Source: TradingSim)
FAQs about Is 100 Trades Enough For Backtesting?
Is 100 trades enough for backtesting?
100 trades may not be sufficient for backtesting as it may not provide a significant sample size to accurately assess the strategy’s performance. There is a risk that the results of the backtesting may not be representative of the strategy’s actual expected performance.
What is the recommended minimum number of trades for backtesting?
The recommended minimum number of trades for backtesting ranges from 30 to 50 trades. However, it ultimately depends on the complexity of the strategy being tested and the level of statistical significance required. A trader may use a larger sample size to increase the confidence levels of the results.
What are the consequences of using too few trades for backtesting?
Using too few trades for backtesting can lead to inaccurate conclusions about the strategy’s expected performance. There is a risk that the results may be overfit to historical data, leading to poor performance in live trading situations. Inadequate sample sizes can also lead to a lack of statistical significance and a higher chance of Type I and Type II errors.
Can I use other methods to supplement my backtesting, even if I only have 100 trades?
Yes, there are other approaches to supplement your backtesting results even if you have only 100 trades. You can conduct walk-forward analysis, forward testing, or Monte Carlo simulations to validate the strategy’s robustness and assess its performance under various market conditions.
What are some best practices for backtesting a strategy?
Some best practices for backtesting a strategy include using a sufficient sample size, incorporating various market conditions, accounting for trading costs, and documenting the backtesting process and results thoroughly. It’s also important to test the strategy on out-of-sample data to ensure it can perform well under new market conditions.
How can I improve my backtesting results if I only have 100 trades?
To improve backtesting results with only 100 trades, a trader should focus on incorporating a wider range of market conditions, use a more extensive range of historical data, and consider using other testing methods, such as forward testing or Monte Carlo simulations. A trader should also ensure that the backtesting process aligns with the strategy’s specific rules and goals.